
Challenges in Operationalizing Responsible AI
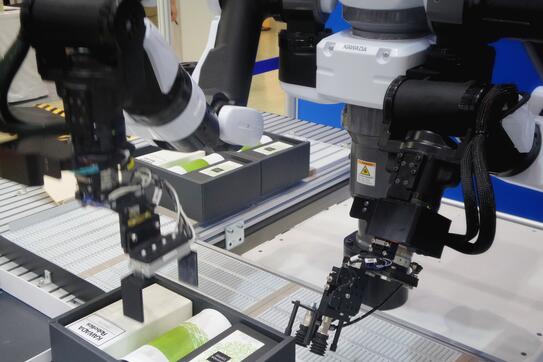
Our Goal
This workshop aims to bring together researchers and practitioners working on the ethical and responsible use of automated algorithms. AI-based decision systems involve several constructed parts, ranging from the gathering of data to its final deployment, and their successful management requires balancing multiple stakeholder interests.
Registration is closed.

Our Focus
We aim to cultivate an open dialogue about operationalizing fairness & robustness considerations, cost management, legal issues, and algorithmic challenges. Our discussions will center around the challenges in putting best practices into action. By bringing together researchers and practitioners across industry and academia, the workshop will be an opportunity to collectively share and reflect on our experiences.

Organized by Hongseok Namkoong
Hongseok Namkoong is an Assistant Professor in the Decision, Risk, and Operations division at Columbia Business School. His research and teaching interests lie at the interface of operations research, machine learning, and statistics.
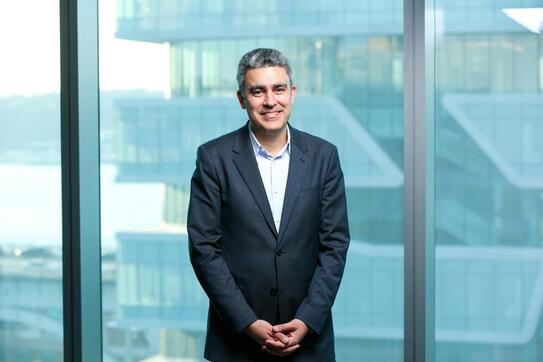
Hosted by Omar Besbes, Digital Future Initiative
Omar Besbes's primary research interests are in the area of data-driven decision-making with a focus on applications in e-commerce, pricing and revenue management, online advertising, operations management and general service systems.
Agenda
8:30am – 9:10am: Registration and Breakfast
9:10am – 9:15am: Opening remarks
9:15am – 10:15am:
- Sharad Goel, Harvard Kennedy
- Eric Talley, Columbia Law School
10:15am – 10:45am: Coffee Break
10:45am - 11:45am:
- John Lewis, Upstart: “Some Considerations for Interpreting Fairness Metrics”
- Sakshi Jain, LinkedIn and Natesh Pillai, LinkedIn/Harvard Statistics
11:45am - 1:00pm: Lunch
1:00pm - 2:00pm:
- Swati Gupta, MIT Sloan: “New Perspectives to Tackle Contextual Biases Across Domains”
- Aleksandra Korolova, Princeton CS: “Platform-supported Auditing of Social Media Algorithms”
2:00pm - 2:45pm: Practitioner’s panel: Operationalizing Responsible AI
- Sakshi Jain, LinkedIn
- John Lewis, Upstart
- Toni Morgan, Tiktok
- Sarah Tan, Cambia Health/Cornell
2:45pm - 3:15pm: Coffee Break
3:15pm - 5pm:
- Lydia Liu, Cornell CS: “Minding the actionability gap of predictions in responsible AI: lessons from education”
- Jiahao Chen, Responsible AI LLC: “Algorithm auditing and risk assessments as tools for AI governance: a field report”
- Tian Wang and Chris McConnell, Meta: “Responsible AI Investment in Meta”
5pm - 5:05pm: Closing remarks
Speakers
Swati Gupta
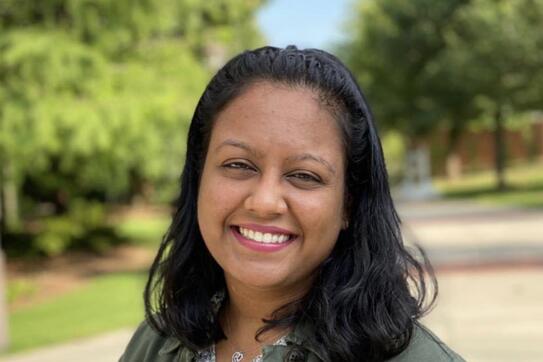
New Perspectives to Tackle Contextual Biases Across Domains
Abstract
Optimization and statistical models based on historical and socio-economic data that do not incorporate fairness desiderata can lead to unfair, discriminatory, or biased outcomes. New ideas are needed to ensure that the developed systems are accountable under uncertainty and reduce a deeper propagation of biases in multi-level decisions. They further need to adhere to various domain-dependent constraints. In this talk, I will highlight our recent work on (i) hiring: how to hire talented individuals without violating anti-discrimination laws when evaluation data embed contextual biases, (ii) admissions: how to reduce segregation in schools using implementable policies despite inherent biases in student data, and (iii) healthcare: how to detect critical conditions using errored electronic medical records. The challenges in each domain are different due to interaction with the law, policy, public perception, and accountability to domain experts, but the solutions exploit the same theoretical principles.
Dr. Swati Gupta is an Assistant Professor at the Sloan School of Management at MIT, in the Operations Research and Statistics Group. Prior to this, she held a Fouts Family Early Career Professorship as an Assistant Professor at the Stewart School of Industrial & Systems Engineering at Georgia Tech. She also served as the lead of Ethical AI in the NSF AI Institute on Advances in Optimization, awarded in 2021. She received a Ph.D. in Operations Research from MIT in 2017, and joint Masters and B.Tech in Computer Science from IIT Delhi in 2011.
Her research interests include optimization and machine learning, with a focus on algorithmic fairness. Her work spans various domains such as hiring, admissions, e-commerce, districting, quantum optimization, and energy. She has received the NSF CAREER Award in 2023, the Class of 1934: Student Recognition of Excellence in Teaching in 2020 and 2021 at Georgia Tech, the JP Morgan Early Career Faculty Award in 2021 and the NSF CISE Research Initiation Initiative Award in 2019. Dr. Gupta’s research is partially funded by the National Science Foundation and DARPA.
Eric Talley

Eric Talley is an expert on the intersection of corporate law, governance, and finance. He additionally teaches and conducts research in the areas of mergers and acquisitions, quantitative methods, machine learning, contract and commercial law, alternative investments, game theory, and economic analysis of law.
As a co-director of the Ira M. Millstein Center for Global Markets and Corporate Ownership, Talley directs research and programs focused on the future of corporate governance and performance. Talley is a frequent commentator in the national media and speaks regularly to corporate boards, judges, and regulators on issues pertaining to fiduciary duties, governance, and finance. He also hosts the Columbia-based podcast, Beyond “Unprecedented”: The Post Pandemic Economy. Talley is a two-time recipient of the Law School’s Willis L.M. Reese Prize for Excellence in Teaching (2017 and 2022).
Lydia T. Liu

Minding the actionability gap of predictions in responsible AI: lessons from education
Abstract
In public policy and social domains, data-driven predictions from ML models are often used as part of an intervention pipeline, or as an intervention itself (e.g. prediction for college and graduate admissions, predictive risk modeling in child welfare services, pre-trial risk assessment tools). Although it is typically assumed that predicting outcomes serves policy interests, we discuss a recent qualitative study with education researchers that questions when developing predictive models is sufficient or necessary for making good interventions. Then, through a graphical model encompassing actions, latent states, and measurements, we demonstrate that pure outcome prediction rarely results in the most effective policy for taking actions, even when combined with other measurements. This talk emphasizes looking beyond outcome prediction and considering downstream interventions in all stages of development as a key step towards responsible AI.
Lydia T. Liu is a postdoctoral researcher at Cornell University, working with Jon Kleinberg, Karen Levy, and Solon Barocas, and incoming assistant professor of Computer Science at Princeton University. Her research examines the theoretical foundations of machine learning and algorithmic decision-making, with a focus on societal impact and human welfare.
She obtained her PhD in Electrical Engineering and Computer Sciences from UC Berkeley, advised by Moritz Hardt and Michael Jordan, and has received a Microsoft Ada Lovelace Fellowship, an Open Philanthropy AI Fellowship, and a Best Paper Award at the International Conference on Machine Learning.
John Lewis
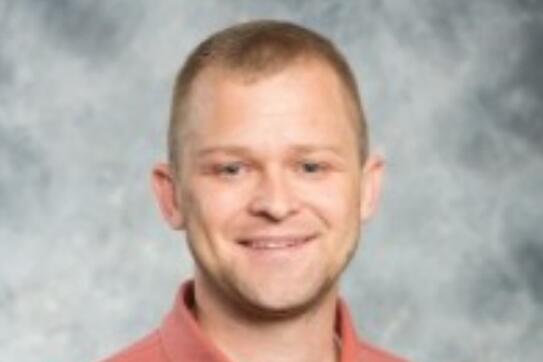
Some Considerations for Interpreting Fairness Metrics
Abstract
Ensuring fairness and explainability in automated credit decisions is of primary importance. First, it helps to foster transparency and build trust among business partners and consumers. Second, there is a legal obligation to comply with regulations like the Equal Credit Opportunity Act Regulation B which define the legal standards related to fairness and explainability in the lending space. In this talk, the focus will be on fairness. Specifically, I will discuss some common metrics used for measuring the fairness of a credit decisioning system and explore considerations when interpreting these metrics.
John Lewis received a PhD in statistics from The Ohio State University in 2014. He worked as a research statistician at Sandia National Laboratories for five years where he did research in various areas including analysis of complex computer models, functional data analysis, space-time models, and inverse problems.
In 2019, John joined Upstart, an AI lending platform, where he worked on developing underwriting models, model governance, and AI fairness related topics. He currently manages the ML Integrity Research group which focuses on model fairness and explainability.
Natesh Pillai

Natesh Pillai is a Distinguished Engineer in LinkedIn and a Professor of Statistics at Harvard University. He obtained his PhD from Duke University in 2008.
He was elected fellow of the Institute of Mathematical Statistics in 2021 and awarded the young researcher award by the International Indian Statistical Association in 2018. His main research interests are computational statistics, reinforcement learning, applied probability, and problems in climate science. He has worked extensively in industry; most recently he was an Amazon scholar.
Tian Wang

Responsible AI Investment in Meta
Abstract
In this talk, we will cover some of Meta's key areas of investment in Responsible AI. In the first part of our talk, we will discuss Meta's current approach to fairness in personalized advertising. We will describe our ongoing efforts to change how ads can be targeted by advertisers and build towards more equitable distribution of ads through our machine learning ads delivery process. In the second part, we will discuss efforts around the transparency of our AI powered recommendation systems, focused on our use of system cards to better communicate with users about the information that we use to make algorithmically-driven decisions on our products.
Tian Wang received his B.S. (2004) degree in physics from the University of Science and Technology of China, the M.Sc. degree in electrical engineering and Ph.D. in physics from North Carolina State University. His main research interest is in modeling and classification of social networks and information flow on social networks.
After graduation, Tian worked for IBM Watson Solution as Staff Software Engineer to apply machine learning in health insurance application and medical research. Since 2015, Tian has been working in Meta as research scientist, specializing in applied machine learning in online digital ads systems. And his current focus is AI Fairness is Ads delivery system.
Jiahao Chen

Algorithm auditing and risk assessments as tools for AI governance: a field report
Abstract
As progress in AI creates new risks for ethical harms, calls for regulations on AI are growing worldwide. The emerging consensus of regulations and frameworks in China, EU, Singapore, and US is the need for algorithmic audits and risk assessments as part of production usage of AI systems. Despite this growing interest in audits as tools for accountability and transparency, there is little consensus in industry on what constitutes an acceptable audit, as well as what to do with the results of an audit.
In this talk, I survey the nascent landscape of algorithmic audits and their coevolution with legislative and regulatory developments across the world. I also report on my industry experience from building industry tools for internal compliance at financial institutions in the USA, as well as building a startup focused on auditing and audit readiness for AI employment tools in New York City. My main findings are: i) gaps in expectations for costs and outcomes of audits, ii) tensions between stakeholders arising from lack of audit preparedness, data availability and transparency expectations, iii) a general need for improved data science practices in algorithmic auditors, and iv) needs to incorporate audit findings into business processes for change management. I conclude with some preliminary thoughts on how we can address the growing pains in the nascent industry of AI governance and risk management, so that the practice of AI in industry can mature as an engineering discipline. (Jiahao Chen (he/him) 陳家豪 (他) is the owner of Responsible AI LLC, which offers algorithmic auditing and other AI governance solutions for enterprises.)
Jiahao Chen is an independent consultant and solopreneur working on responsible AI (RAI), with a focus on global AI governance and risk management. His clients span the gamut of private companies, nonprofits, and government agencies. His consulting connects him with diverse client needs, which directly drives his product roadmap for his B2B SaaS startup to handle diverse user needs for RAI across different verticals and jurisdictions.
With private clients, he specializes in developing enterprise-ready solutions for developing responsible AI systems in highly regulated industries such as financial services, employment, and national security, taking appropriate global perspectives in AI regulation across the US, UK, EU, Singapore, China, and other jurisdictions. He helps clients develop business strategies to connect qualitative ethical reasoning to quantitative implementation and concrete processes for development, testing and evaluation. He remains active in academic research and is published in leading venues (Google Scholar). His current work with nonprofits includes advising on open source development, investigative data journalism, and being an Ethics Chair of the NeurIPS.
Sarah Tan

Sarah Tan (Cambia Health / Cornell University) is an AI Scientist at Cambia Health Solutions, leading work on responsible AI and large language models. Cambia Health Solutions operates Blue Cross Blue Shield (BCBS) in 4 states and is part of the BCBS Association, a collection of BCBS companies that collectively provide health insurance to more than 100 million Americans. Before Cambia, she worked at Facebook in Responsible AI, and also worked in public policy in NYC, including the health department and public hospitals system. She is also a Visiting Scientist at Cornell University and President of the Women in Machine Learning nonprofit. She received her PhD from Cornell University, and was recently Tutorial Chair at the FAccT 2023 conference.
Aleksandra Korolova

Platform-supported Auditing of Social Media Algorithms
Abstract
Social media platforms curate access to information and opportunities, and so play a critical role in shaping public discourse. The opaque nature of the relevance-estimator algorithms these platforms use to curate content raises societal questions. Prior studies have used black-box methods led by experts or collaborative audits driven by everyday users to show that these algorithms can lead to discriminatory outcomes. However, existing auditing methods face fundamental limitations. We propose a novel platform-supported framework to allow researchers to audit relevance-estimator algorithms. The framework gives auditors privileged query-access to platforms’ relevance estimators in a way that allows auditing for bias in the algorithms while preserving the privacy interests of users and platforms. Our technical contributions, combined with ongoing legal and policy efforts, can enable public oversight into how social media platforms affect individuals and society by moving past the often-cited privacy-vs-transparency hurdle. Joint work with Basileal Imana and John Heidemann.
Aleksandra Korolova is an Assistant Professor of Computer Science and Public Affairs at Princeton and a member of Princeton's Center for Information Technology Policy. She studies the societal impacts of algorithms and machine learning and develops and deploys algorithms that enable data-driven innovations while preserving privacy and fairness. She also designs and performs algorithm and AI audits.
Aleksandra is a recipient of the 2020 NSF CAREER award, a co-winner of the 2011 PET Award for outstanding research in privacy enhancing technologies for exposing privacy violations of microtargeted advertising and a runner-up for the 2015 PET Award for RAPPOR, the first commercial deployment of differential privacy. Aleksandra's most recent research, on discrimination in ad delivery, has received the 2019 CSCW Honorable Mention Award and Recognition of Contribution to Diversity and Inclusion and was a runner-up for the 2021 WWW Best Student Paper Award. Prior to joining Princeton, Aleksandra was a WiSE Gabilan Assistant Professor of Computer Science at USC, a Privacy Advisor at Snap and a Research Scientist at Google.
Sharad Goel
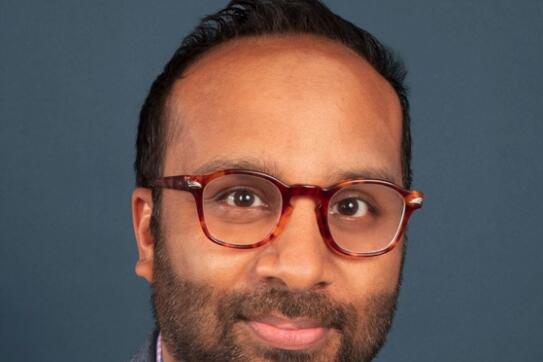
Sharad Goel is a Professor of Public Policy at Harvard Kennedy School. He looks at public policy through the lens of computer science, bringing a computational perspective to a diverse range of contemporary social and political issues, including criminal justice reform, democratic governance, and the equitable design of algorithms. Prior to joining Harvard, Sharad was on the faculty at Stanford University, with appointments in management science & engineering, computer science, sociology, and the law school. He holds an undergraduate degree in mathematics from the University of Chicago, as well as a master’s degree in computer science and a doctorate in applied mathematics from Cornell University.
Chris McConnell

Chris McConnell is a Research Scientist on Meta's Responsible AI team. He has worked on several topics within RAI, including fairness in ranking problems. His current interests include the long-term impact of fairness interventions in two-sided recommendation systems and incorporating strategic user behavior into the study of algorithmic fairness. He is originally from Charlottesville, Virginia.
Sakshi Jain

Sakshi Jain leads the Responsible AI efforts within Data at LinkedIn, which focuses on making LinkedIn products fair, transparent and privacy-sensitive. She graduated from UC Berkeley with Prof. David Wagner & Prof. Vern Paxon working at the intersection of Machine Learning and Network Security. She spent 7 years building AI based defenses against large scale adversarial attacks on the platform before setting up the Responsible AI team within LinkedIn.
Toni Morgan
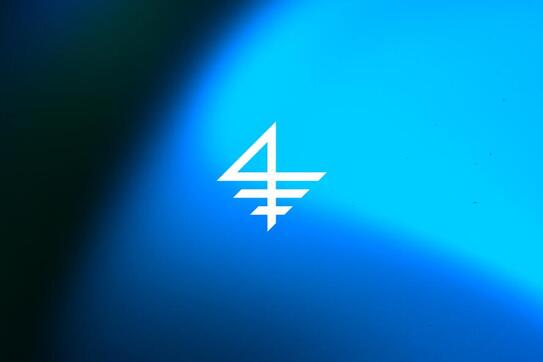